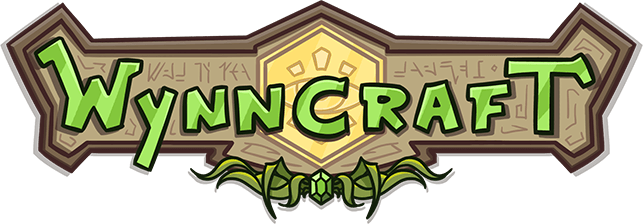
Dismiss Notice

Wynncraft, the Minecraft MMORPG. Play it now on your Minecraft client at (IP): play.wynncraft.com. No mods required! Click here for more info...
Guide 2.0 Forgery Odds And You: A Mathematical Analysis
Discussion in 'Wynncraft' started by Sockmower, Sep 5, 2022.
Tags:
- Thread Status:
- Not open for further replies.
- Thread Status:
- Not open for further replies.